Statistical Machine Learning
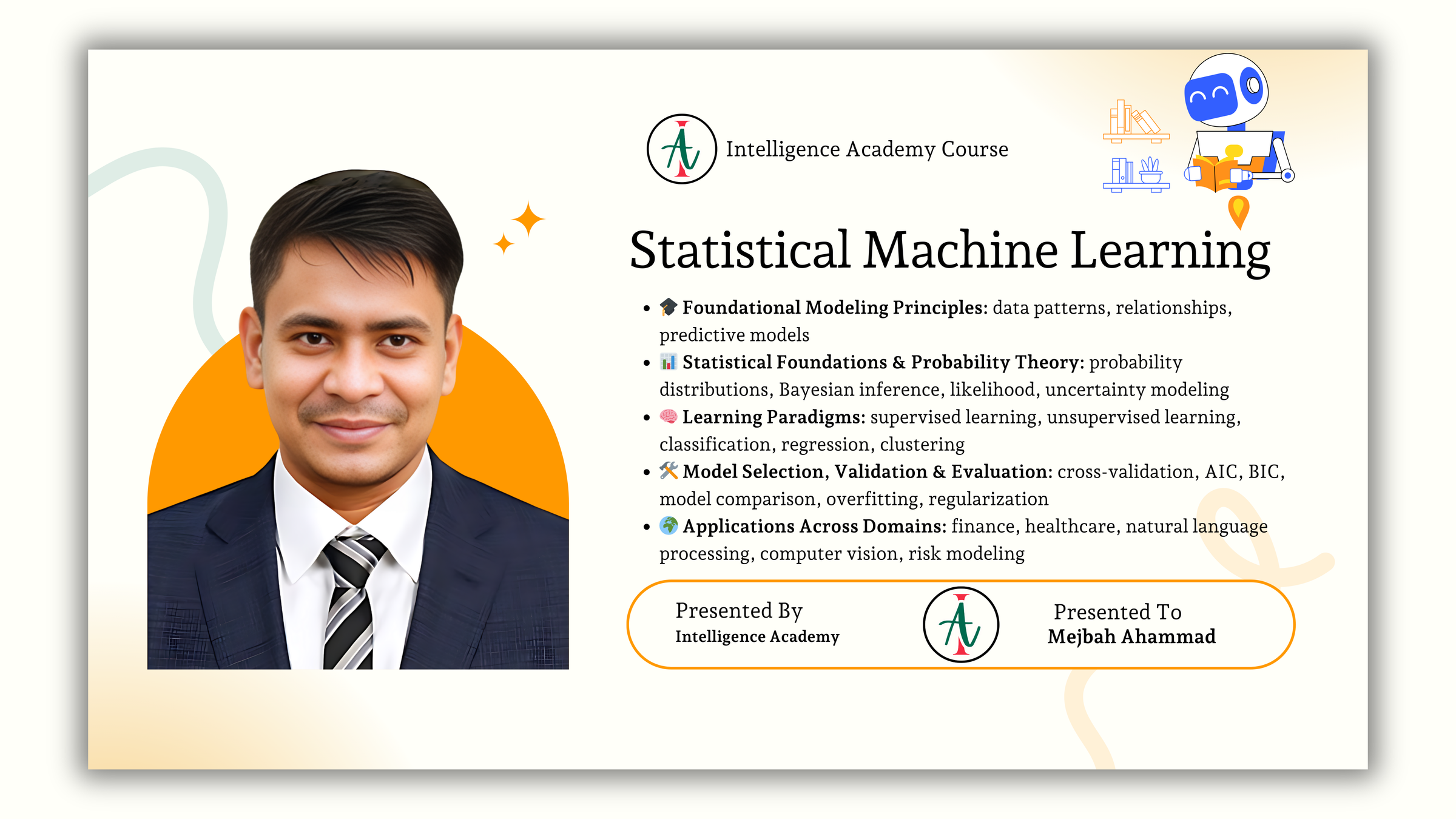
1. Overview & Logistics
- ๐จโ๐ซ Instructor: Mejbah Ahammad
- ๐๏ธ Semester: Spring Semester
- โฐ Class Time: 8:00 PM โ 10:00 PM
- ๐ Class Days: Saturday and Wednesday
- ๐ป Class Mode: Remote (Zoom)
- ๐ฐ Course Fee: เงณ4000
- โ๏ธ Contact Number: +8801874603631
- โ Lessons & Time: 20 Lessons, 40 เฆเฆจเงเฆเฆพ 20 เฆฎเฆฟเฆจเฆฟเฆ total
- ๐ง Email: hello@softwareintelligence.ai
- ๐ Website: http://softwareintelligence.ai/
2. Course Description
Statistical Machine Learning combines:
- ๐ Statistical Foundations: Probability, distributions, estimation, hypothesis testing
- ๐ Machine Learning Techniques: Regression, classification, clustering, ensemble methods
- ๐ป Practical Coding: Python (
NumPy
,pandas
,scikit-learn
) for model building and evaluation - ๐ Advanced Topics: Bayesian inference, neural networks, SVMs, interpretability, ethics
- ๐ฃ๏ธ Communication: Presentation, reporting, real-world problem-solving
By course end, participants will build robust ML pipelines using statistical rigor and cutting-edge techniques, culminating in a capstone-style final project or demonstration.
3. Learning Outcomes
-
๐ Foundational Skills (Beginner)
- ๐ Recognize basic probability concepts, distributions, and inference methods.
- ๐ Perform initial data cleaning, wrangling, and EDA in Python.
-
๐ Intermediate Skills
- ๐ Develop supervised ML models (linear/logistic regression, ensembles).
- ๐ Understand regularization, model tuning, and cross-validation strategies.
-
๐ก Advanced Skills
- ๐ Implement Bayesian methods, SVMs, neural networks, or advanced ensemble strategies.
- ๐ Critically analyze model assumptions, interpret results, and address ethical concerns.
-
๐ฃ๏ธ Professional Communication
- ๐ Present findings to diverse audiences with clarity (visuals, reports).
- ๐ Collaborate effectively, incorporating peer/instructor feedback.
4. Prerequisites
-
๐ Mathematics & Statistics:
- Basic probability (Normal, Binomial), inferential stats (t-tests, p-values).
- Some exposure to linear algebra (matrix operations) and calculus (derivatives).
-
๐ป Programming:
- Familiarity with Python (lists, loops, functions).
- Basic usage of
pandas
,NumPy
,matplotlib
, or similar.
-
๐ผ Logistics & Tools:
- Stable internet for Zoom.
- Python environment (Anaconda recommended).
- Willingness to install additional Python packages (e.g.,
scikit-learn
).
5. Course Materials
A. Required Texts/Readings
- ๐ An Introduction to Statistical Learning (ISL) by James, Witten, Hastie, Tibshirani (Springer).
- ๐ The Elements of Statistical Learning (ESL) by Hastie, Tibshirani, Friedman (Springer).
B. Recommended
- ๐ Pattern Recognition and Machine Learning by Christopher Bishop (Springer).
- ๐ Bayesian Data Analysis by Gelman et al. (CRC Press).
- Official Python & scikit-learn documentation.
C. Software
- ๐ป Python 3.x (Anaconda)
- ๐ Jupyter Notebook / IDE (VSCode, PyCharm)
- ๐ฅ๏ธ Zoom for remote sessions
6. Schedule & Lessons (20 Classes, 40 Hours 20 Minutes)
Each lesson is designed for approximately 2 hours (some might slightly exceed to total 40:20). Classes blend theory and hands-on demos or exercises.
Lesson | Topic | Level | Key Focus |
---|---|---|---|
1 | ๐ Course Introduction & Probability Review | Beginner | Syllabus, environment setup, distributions (Bernoulli, Normal), random variables |
2 | ๐ Parameter Estimation (MLE & MAP) | Beginner โ Intermediate | Likelihood functions, Bayesian vs. frequentist approaches, small coding demos |
3 | ๐ Exploratory Data Analysis & Hypothesis Testing | Beginner โ Intermediate | Data wrangling, missing values, EDA, t-tests, p-values, confidence intervals |
4 | ๐ Linear Regression & Regularization | Intermediate | OLS, Ridge, Lasso, cross-validation, bias-variance trade-off |
5 | ๐ Logistic Regression & Classification Metrics | Intermediate | Confusion matrix, precision/recall, ROC-AUC, cross-entropy |
6 | ๐ Feature Engineering & Model Diagnostics | Intermediate | Categorical encoding, polynomial features, residual analysis, error estimation |
7 | ๐ Bayesian Methods & Conjugate Priors | Intermediate โ Advanced | Posterior updates, Beta-Bernoulli, Normal-Normal, MCMC basics |
8 | ๐ Decision Trees & Ensemble Methods (Bagging, RF) | Intermediate โ Advanced | CART, random forests, OOB errors, feature importance, bagging strategies |
9 | ๐ Boosting & Advanced Ensemble (AdaBoost, XGBoost) | Intermediate โ Advanced | Gradient boosting, sequential error correction, hyperparameter tuning |
10 | ๐ Support Vector Machines (Foundations) | Intermediate โ Advanced | Margin maximization, kernel trick, soft/hard margins |
11 | ๐ SVM in Practice & Tuning | Advanced | RBF, polynomial kernels, grid/random search, practical pitfalls |
12 | ๐ Dimensionality Reduction (PCA, LDA) | Intermediate โ Advanced | Covariance, eigen-decomposition, linear discriminants, advanced manifold methods (optional) |
13 | ๐ Clustering (K-means, Hierarchical, DBSCAN) | Intermediate โ Advanced | Unsupervised basics, cluster validation (silhouette), dendrograms, density-based methods |
14 | ๐ Probabilistic Graphical Models (Bayesian Networks) | Advanced | Conditional independence, factorization, small examples, potential software for inference |
15 | ๐ Hidden Markov Models & Sequential Data | Advanced | Markov chains, forward-backward algorithm, Viterbi decoding, time-series aspects |
16 | ๐ Neural Networks (MLP Intro) | Advanced | Perceptron, activation functions, backprop basics, capacity vs. data requirements |
7. Detailed Lesson Descriptions
Lesson 1 (2 Hours)
๐ Topic: Course Introduction & Probability Review
- ๐ Focus: Syllabus overview, environment check, distributions (Bernoulli, Normal), sampling basics
- ๐ Assignment:
- Install libraries (NumPy, pandas, scikit-learn).
- Short quiz on probability concepts.
- ๐ผ Professional Insight:
- Probability underpins risk modeling (finance, insurance) and quality control (manufacturing).
- Proper environment setup mirrors DevOps best practices for reproducible data science.
Lesson 2 (2 Hours)
๐ Topic: Parameter Estimation (MLE & MAP)
- ๐ Focus: Likelihood functions, frequentist vs. Bayesian viewpoint, small coding demos
- ๐ Assignment:
- Compare MLE and MAP estimates on a simple dataset (e.g., coin toss).
- ๐ผ Professional Insight:
- MLE vs. MAP is critical in marketing analytics (conversion rates) or medical testing (disease prevalence).
- Choosing an appropriate prior (MAP) can incorporate domain knowledge in real-world deployments.
Lesson 3 (2 Hours)
๐ Topic: Exploratory Data Analysis & Hypothesis Testing
- ๐ Focus: Data wrangling, missing value handling, outlier detection, t-tests, p-values
- ๐ Assignment:
- Clean a real dataset, run basic hypothesis tests (A/B style).
- ๐ผ Professional Insight:
- EDA is ~80% of real data science tasks. Quick hypothesis tests guide business decisions (new product vs. old).
- Communicating results in non-technical terms fosters stakeholder trust.
Lesson 4 (2 Hours)
๐ Topic: Linear Regression & Regularization (Ridge, Lasso)
- ๐ Focus: OLS assumptions, bias-variance, cross-validation, controlling overfitting
- ๐ Assignment:
- Compare OLS vs. Ridge vs. Lasso on a regression problem (e.g., housing).
- ๐ผ Professional Insight:
- Common approach for pricing strategy, sales forecasting, and resource planning.
- Regularization ensures stability in production, saving compute costs by preventing overfitting.
Lesson 5 (2 Hours)
๐ Topic: Logistic Regression & Classification Metrics
- ๐ Focus: Confusion matrix, precision/recall, ROC-AUC, threshold tuning
- ๐ Assignment:
- Classify Titanic-like data; interpret different metrics.
- ๐ผ Professional Insight:
- Logistic regression is key in credit scoring, churn prediction, and medical diagnosis.
- Understanding metrics aligns models with business objectives (precision vs. recall trade-offs).
Lesson 6 (2 Hours)
๐ Topic: Feature Engineering & Model Diagnostics
- ๐ Focus: Encoding categorical variables, polynomial transformations, residual/error analysis
- ๐ Assignment:
- Enhance feature set, compare performance gains, analyze errors thoroughly.
- ๐ผ Professional Insight:
- In real-world ML, feature engineering often trumps fancy algorithms in terms of improvement.
- Detailed error analysis helps refine future data collection and domain strategies.
Lesson 7 (2 Hours)
๐ Topic: Bayesian Methods & Conjugate Priors
- ๐ Focus: Posterior derivation, Beta-Bernoulli, Normal-Normal, intro to MCMC tools
- ๐ Assignment:
- Perform Bayesian inference on a small dataset; compare to frequentist results.
- ๐ผ Professional Insight:
- Bayesian methods handle low-data or high-uncertainty environments (startups, medical research).
- MCMC used in complex risk modeling (insurance, environmental studies).
Lesson 8 (2 Hours)
๐ Topic: Decision Trees & Ensemble Methods (Bagging, RF)
- ๐ Focus: CART, random forests, OOB errors, feature importance
- ๐ Assignment:
- Fit a decision tree & a random forest, compare error rates & interpret features.
- ๐ผ Professional Insight:
- Random forests are widely used in finance, healthcare, e-commerce for their interpretability & performance.
- Bagging strategies often reduce variance in high-stakes fields (credit risk, fraud detection).
Lesson 9 (2 Hours)
๐ Topic: Boosting & Advanced Ensemble (AdaBoost, XGBoost)
- ๐ Focus: Sequential error correction, gradient boosting, hyperparameter tuning
- ๐ Assignment:
- Evaluate AdaBoost vs. XGBoost on a classification/regression dataset; tune parameters.
- ๐ผ Professional Insight:
- XGBoost dominates Kaggle competitions & corporate environments (marketing, sales forecasting).
- Boosting algorithms can quickly overfit if not carefully tunedโan important skill in production ML.
Lesson 10 (2 Hours)
๐ Topic: Support Vector Machines (Foundations)
- ๐ Focus: Margin maximization, kernel trick, soft/hard margins
- ๐ Assignment:
- Implement SVM on a 2D classification problem, visualize decision boundaries.
- ๐ผ Professional Insight:
- SVMs excel in high-dimensional data (text classification, genetics).
- Understanding kernel selection is vital for certain image or speech tasks.
Lesson 11 (2 Hours)
๐ Topic: SVM in Practice & Tuning
- ๐ Focus: RBF, polynomial kernels, grid/random search, practical pitfalls
- ๐ Assignment:
- Use
GridSearchCV
to tune hyperparameters (C, gamma) on a real dataset.
- Use
- ๐ผ Professional Insight:
- Proper parameter tuning can drastically shift model accuracy.
- SVM remains a strong baseline in many industrial AI solutions.
Lesson 12 (2 Hours)
๐ Topic: Dimensionality Reduction (PCA, LDA)
- ๐ Focus: Covariance, eigen-decomposition, supervised vs. unsupervised dimension reduction
- ๐ Assignment:
- Apply PCA to a high-dimensional dataset; optionally compare LDA for classification.
- ๐ผ Professional Insight:
- Reducing dimensionality helps in visualization and speed for real-time applications (IoT, sensor data).
- LDA commonly used in face recognition, medical classification tasks.
Lesson 13 (2 Hours)
๐ Topic: Clustering (K-means, Hierarchical, DBSCAN)
- ๐ Focus: Unsupervised basics, cluster validation, dendrograms, density-based algorithms
- ๐ Assignment:
- Compare at least two clustering methods, interpret results with silhouette scores.
- ๐ผ Professional Insight:
- Clustering widely used in customer segmentation, market research, and anomaly detection.
- DBSCAN or hierarchical clustering can reveal irregular cluster structures in real data.
Lesson 14 (2 Hours)
๐ Topic: Probabilistic Graphical Models (Bayesian Networks)
- ๐ Focus: Graph structures, conditional independence, small examples
- ๐ Assignment:
- Construct or analyze a simple Bayesian network; perform basic inference.
- ๐ผ Professional Insight:
- Graphical models appear in medical diagnosis (causal inference), sensor fusion, and complex decision-making.
- Visualizing dependencies helps stakeholders grasp complicated relationships.
Lesson 15 (2 Hours)
๐ Topic: Hidden Markov Models & Sequential Data
- ๐ Focus: Markov chains, forward-backward, Viterbi decoding, possible link to time series
- ๐ Assignment:
- Implement an HMM for a toy sequence (e.g., weather states or text).
- ๐ผ Professional Insight:
- HMMs are cornerstones in speech recognition, bioinformatics (DNA sequences), and POS tagging in NLP.
- Sequential modeling is crucial in many real-time or streaming applications.
Lesson 16 (2 Hours)
๐ Topic: Neural Networks (MLP Intro)
- ๐ Focus: Perceptron/MLP basics, activation functions, high-level backprop
- ๐ Assignment:
- Train a small MLP on a classification dataset, discuss overfitting.
- ๐ผ Professional Insight:
- Neural nets power advanced computer vision and NLP tasks in top tech companies.
- Balancing model complexity vs. data is critical in production cost management.
Lesson 17 (2 Hours)
๐ Topic: Overfitting & Robust Validation
- ๐ Focus: Dropout (in neural nets), early stopping, nested CV, data augmentation
- ๐ Assignment:
- Show how advanced validation and regularization reduce overfitting in a prior model.
- ๐ผ Professional Insight:
- Overfitting leads to financial losses (poor predictions) or misdiagnoses (healthcare).
- Rigorous validation fosters trust and reliability in deployed ML systems.
Lesson 18 (2 Hours)
๐ Topic: Interpretability & Fairness in ML
- ๐ Focus: Tools (LIME, SHAP), fairness and bias, ethical frameworks (GDPR)
- ๐ Assignment:
- Analyze model outputs with SHAP on a selected dataset; discuss potential biases.
- ๐ผ Professional Insight:
- Explainable AI is increasingly required in regulated sectors (finance, healthcare).
- Addressing bias fosters equitable and responsible AI solutions.
Lesson 19 (2 Hours)
๐ Topic: Capstone Project Workshop
- ๐ Focus: Data selection, model design, refining scope, peer/instructor Q&A
- ๐ Assignment:
- Prepare a prototype or outline for your final project.
- ๐ผ Professional Insight:
- Mimics team stand-ups or project reviews in corporate data science.
- Early feedback loop ensures agile methodology and timely pivots.
Lesson 20 (2 Hours + 20 mins)
๐ Topic: Capstone Presentations & Course Wrap-Up
- ๐ Focus: Student/Team presentations, Q&A, advanced resources, next steps in ML
- ๐ Deliverable:
- Final code/report + demonstration.
- Course feedback or survey.
- ๐ผ Professional Insight:
- Polished presentations simulate pitching to executives or clients.
- Reflecting on advanced directions (deep learning frameworks, big data) fosters continual growth.
8. Assessment & Grading
-
๐ Weekly/Regular Assignments (40%)
- ๐ Coding tasks, problem-solving, reflection papers.
- Reinforces theory with hands-on practice.
-
๐ Quizzes (10%)
- ๐ Short checks on stats & ML fundamentals (announced or pop).
- Encourages consistent revision.
-
๐ผ Capstone Project (40%)
- ๐ End-to-end ML pipeline: data prep โ modeling โ validation โ interpretability โ presentation.
- Demonstrates integrated skills from the entire course.
-
๐ค Participation (10%)
- ๐ Active engagement, Q&A, breakout rooms, peer feedback.
- Collaboration skill is essential in real-world DS teams.
๐ท๏ธ Grade Scale
- A = 90โ100%
- B = 80โ89%
- C = 70โ79%
- D = 60โ69%
- F = < 60%
9. Course Policies
-
๐ท๏ธ Attendance & Engagement
- Mandatory Zoom attendance (camera on recommended).
- Inform absences in advance if possible.
-
๐ข Communication
- Check email regularly for announcements.
- Email hello@softwareintelligence.ai for questions or clarifications.
-
โฒ๏ธ Late Submissions
- May incur penalties unless pre-approved.
- Discuss extensions for valid reasons (health, emergencies).
-
โ ๏ธ Academic Integrity
- No plagiarism or unapproved collaboration.
- Violations follow institutional guidelines.
-
๐ป Technical Setup
- Install/maintain Python environment (Anaconda).
- Ensure stable internet, Zoom readiness.
10. Final Note
Welcome to Statistical Machine Learning! Over 20 Lessons (total 40 hours 20 minutes), expect an interactive deep dive into stats + ML. Keep in mind:
- Practice consistently with real datasets.
- Engage with peers for feedback and troubleshooting.
- Document your processesโtransparency is key to professional data science.
We look forward to a dynamic, hands-on semester together!
๐จโ๐ซ Instructor: Mejbah Ahammad
โ๏ธ Phone: +8801874603631
๐ Website: http://softwareintelligence.ai/
๐ง Email: hello@softwareintelligence.ai
(C) 2025 Software Intelligence & Intelligence Academy โ All Rights Reserved.