Mastering in Image Processing and Computer Vision
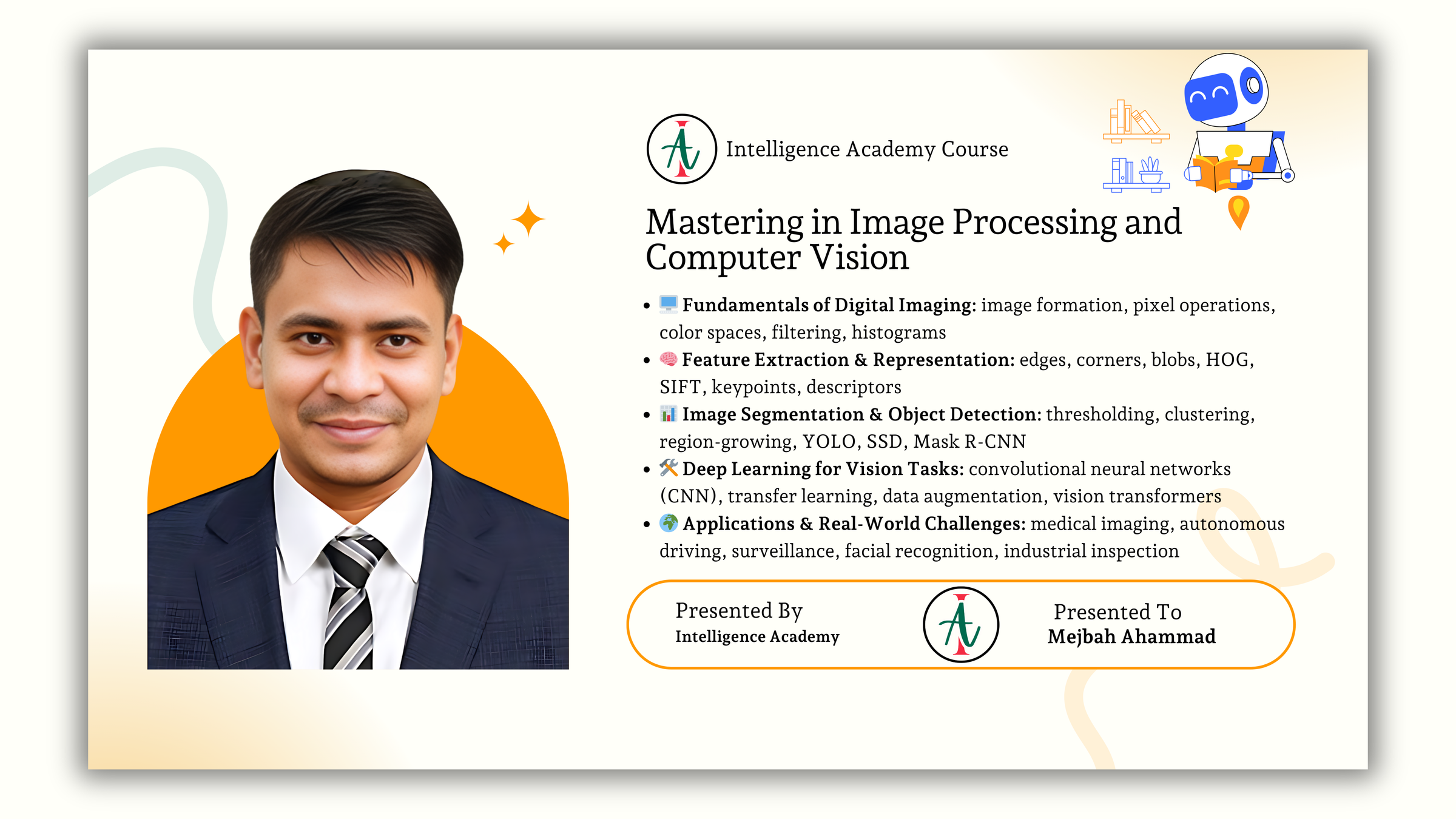
1. Overview & Logistics
- ๐จโ๐ซ Instructor: Mejbah Ahammad
- ๐๏ธ Semester: Spring Semester
- โฐ Class Time: 8:00 PM โ 10:00 PM
- ๐ Class Days: Sunday and Thursday
- ๐ป Class Mode: Remote (Zoom)
- ๐ฐ Course Fee: เงณ4000
- โ๏ธ Contact Number: +8801874603631
- โ Lessons & Time: 20 Lessons, 40 เฆเฆจเงเฆเฆพ 20 เฆฎเฆฟเฆจเฆฟเฆ total
- ๐ง Email: hello@softwareintelligence.ai
- ๐ Website: http://softwareintelligence.ai/
2. Course Description
Mastering in Image Processing and Computer Vision aims to provide:
- ๐ด Fundamentals of Image Processing: Image representation, color spaces, filtering, frequency domain methods
- ๐ต Feature Extraction & Object Recognition: Keypoint detectors, machine learning classification, deep learning (CNNs)
- ๐ Advanced Topics: 3D vision, medical imaging, GANs, vision transformers, AI ethics
- ๐ข Research & Real-World Applications: SLAM for autonomous vehicles, final project, domain-specific explorations
By the end, participants will build robust computer vision pipelines that integrate classic image processing and state-of-the-art deep learning solutions, culminating in a capstone project or research-driven demonstration.
3. Learning Outcomes
-
๐ Foundational Skills (Beginner)
- ๐ Describe image fundamentals (color models, resolution).
- ๐ Implement basic filters, morphological ops, and transformations in Python.
-
๐ Intermediate Skills
- ๐ Extract and match features (SIFT, SURF, ORB), build ML classification pipelines.
- ๐ Perform object detection (HOG+SVM, Haar) and tracking (Kalman filter, Mean-Shift).
-
๐ Advanced Skills
- ๐ Develop deep learning solutions (CNNs, GANs, segmentation networks, ViTs).
- ๐ Tackle 3D reconstructions, SLAM, and specialized tasks (medical imaging, domain adaptation).
-
๐ฃ๏ธ Professional Communication
- ๐ Present final projects with clarity (demos, dashboards).
- ๐ Draft research reports, prepare for publications or advanced study.
4. Prerequisites
-
๐ Mathematics & Linear Algebra
- Familiarity with matrix operations, eigenvectors, basic probability (Gaussian distributions).
-
๐ป Programming
- Proficiency in Python (lists, loops, functions).
- Some exposure to OpenCV, NumPy, matplotlib, or equivalent.
-
๐ผ Logistics & Tools
- Stable internet connection for Zoom.
- Python environment (Anaconda recommended).
- Willingness to install frameworks (TensorFlow/PyTorch, etc.).
5. Course Materials
A. Required Texts/Readings
- ๐ Digital Image Processing โ Gonzalez & Woods
- ๐ Computer Vision: Algorithms and Applications โ Richard Szeliski
B. Recommended
- ๐ Deep Learning for Vision โ Ian Goodfellow
- Research Papers & Tutorials (e.g., for GANs, Vision Transformers)
- Official OpenCV documentation
C. Software
- ๐ป Python 3.x (Anaconda)
- ๐ Jupyter Notebook / IDE (VSCode, PyCharm)
- ๐ฅ๏ธ Zoom for remote sessions
6. Schedule & Lessons (20 Classes, 40 Hours 20 Minutes)
Lesson | Topic | Level | Key Focus |
---|---|---|---|
1 | ๐ Digital Image Representation & Color Spaces | Beginner | Pixels, color models (RGB, HSV, YUV), bit depth, compression (JPEG, PNG) |
2 | ๐ Mathematical Foundations for Image Processing | Beginner โ Intermediate | Linear algebra (matrices, eigenvectors), probability (Gaussian), convolution, filtering |
3 | ๐ Geometric Transformations & Image Warping | Beginner โ Intermediate | Translation, scaling, rotation, affine/perspective transforms, homography, stitching |
4 | ๐ Spatial & Frequency Domain Processing | Intermediate | Gaussian/median/bilateral filters, Fourier transform (DFT/FFT), DCT in compression |
5 | ๐ Edge Detection & Image Segmentation | Intermediate | Sobel/Canny edges, thresholding (Otsu, adaptive), watershed, graph cuts |
6 | ๐ Morphological Image Processing | Intermediate | Erosion, dilation, opening/closing, skeletonization, connected components |
7 | ๐ Feature Detection & Extraction | Intermediate โ Advanced | Harris, FAST corners, SIFT/SURF/ORB, HOG descriptors |
8 | ๐ Feature Matching & Object Recognition | Intermediate โ Advanced | Brute-Force/FLANN matching, Bag of Visual Words (BoVW), template matching |
9 | ๐ Machine Learning for Image Classification | Intermediate โ Advanced | SVM, k-NN, Decision Trees, PCA & LDA for feature reduction, feature engineering |
10 | ๐ Deep Learning for Image Processing | Intermediate โ Advanced | CNN fundamentals, transfer learning (ResNet, MobileNet), implementation in TensorFlow/PyTorch |
11 | ๐ Object Detection & Tracking | Advanced | Haar, HOG+SVM, YOLO/SSD/Faster R-CNN, tracking (Kalman, Mean-Shift, DeepSORT) |
12 | ๐ Optical Flow & Motion Analysis | Advanced | Lucas-Kanade, Farneback optical flow, background subtraction (MOG2, KNN), surveillance & autonomous vehicles |
13 | ๐ 3D Computer Vision & Depth Estimation | Advanced | Stereo vision, disparity mapping, Structure from Motion (SfM), 3D reconstruction |
14 | ๐ Neural Networks for Image Segmentation | Advanced | Semantic segmentation (UNet, DeepLab), instance segmentation (Mask R-CNN), medical imaging applications |
๐ด MODULE 1: Fundamentals of Image Processing (Classes 1โ6)
Class 1: Digital Image Representation & Color Spaces
- ๐ Key Topics: Pixels, resolution, bit depth; color models (RGB, HSV, YUV, YCbCr); image compression (JPEG, PNG)
- ๐ Assignment: Load images in Python/OpenCV; compare color models, analyze compression artifacts.
- ๐ผ Professional Insight:
- Understanding color transformations is vital in printing, photography, and industrial QA for color consistency.
- Compression trade-offs matter in web streaming, medical imaging, and archiving.
Class 2: Mathematical Foundations for Image Processing
- ๐ Key Topics: Linear algebra (matrices, eigenvectors, PCA), probability & Gaussian distributions, convolutions
- ๐ Assignment: Implement kernel operations (blur, sharpen), test PCA-based dimensionality reduction on images.
- ๐ผ Professional Insight:
- Convolution underlies advanced ML (CNNs).
- PCA helps in data compression or speed-ups for real-time systems.
Class 3: Geometric Transformations & Image Warping
- ๐ Key Topics: Translation, scaling, rotation, affine/perspective transforms, homography & warping
- ๐ Assignment: Create a panorama by stitching images using homography.
- ๐ผ Professional Insight:
- Used in augmented reality for planar object overlays, drone or satellite image alignment.
Class 4: Spatial & Frequency Domain Processing
- ๐ Key Topics: Gaussian/median/bilateral filters, Fourier Transform (DFT/FFT), DCT in compression
- ๐ Assignment: Filter noisy images in frequency domain; explore DCT-based compression effects.
- ๐ผ Professional Insight:
- Frequency domain filtering helps remove periodic noise (e.g., camera flicker).
- DCT is core to JPEGโcrucial for any web-based or mobile imaging pipeline.
Class 5: Edge Detection & Image Segmentation
- ๐ Key Topics: Sobel/Prewitt/Canny edge detection, thresholding (Otsu, adaptive), watershed/graph cuts
- ๐ Assignment: Implement Canny edges, segment images with watershed or graph cuts.
- ๐ผ Professional Insight:
- Edge detection is fundamental for barcode/Qr scanning, contour-based object detection.
- Segmentation is key in medical (tumor boundary), agriculture (plant region), and industrial (defect detection).
Class 6: Morphological Image Processing
- ๐ Key Topics: Erosion, dilation, opening, closing, convex hull, skeletonization, connected components
- ๐ Assignment: Apply morphological ops to separate objects, compute shape descriptors.
- ๐ผ Professional Insight:
- Morphological transformations are used in document analysis (noise removal in scanned text) and factory automation (closing small gaps on part outlines).
๐ต MODULE 2: Feature Extraction & Object Recognition (Classes 7โ12)
Class 7: Feature Detection & Extraction
- ๐ Key Topics: Corner detection (Harris, FAST), SIFT, SURF, ORB, BRIEF, FREAK, HOG
- ๐ Assignment: Compare feature detectors on an image set (speed vs. accuracy).
- ๐ผ Professional Insight:
- Feature points drive SLAM in robotics, marker-based AR, and 2Dโ3D reconstructions.
Class 8: Feature Matching & Object Recognition
- ๐ Key Topics: Brute-force matching (BFMatcher), FLANN, Bag of Visual Words (BoVW), template matching
- ๐ Assignment: Implement a small-scale object recognition (e.g., logo detection) with BoVW or template matching.
- ๐ผ Professional Insight:
- Key approach for retail (product logo recognition), industrial robotics (part detection), and security (symbol detection).
Class 9: Machine Learning for Image Classification
- ๐ Key Topics: Traditional ML (SVM, k-NN, Decision Trees), PCA & LDA for feature reduction, feature engineering
- ๐ Assignment: Build an SVM classifier on a small dataset; compare PCA- vs. LDA-based dimension reductions.
- ๐ผ Professional Insight:
- Traditional ML often suffices in resource-limited or smaller-scale applications.
- PCA/LDA reduce computational overhead in mobile/edge devices.
Class 10: Deep Learning for Image Processing
- ๐ Key Topics: Convolutional Neural Networks (CNNs), transfer learning (ResNet, MobileNet), implementation in TF/PyTorch
- ๐ Assignment: Fine-tune a pre-trained CNN for a custom dataset (e.g., classification or simple detection).
- ๐ผ Professional Insight:
- CNN-based solutions dominate state-of-the-art in recognition tasks (ImageNet benchmarks).
- Transfer learning drastically cuts down training time and data requirements.
Class 11: Object Detection & Tracking
- ๐ Key Topics: Haar Cascades, HOG+SVM, YOLO/SSD/Faster R-CNN, tracking (Kalman Filter, Mean-Shift, DeepSORT)
- ๐ Assignment: Real-time detection + tracking pipeline on video; measure FPS, accuracy.
- ๐ผ Professional Insight:
- Essential in surveillance (people/vehicle tracking), retail analytics, self-driving test rigs.
Class 12: Optical Flow & Motion Analysis
- ๐ Key Topics: Lucas-Kanade, Farneback optical flow, background subtraction (MOG2, KNN), surveillance/autonomous vehicles
- ๐ Assignment: Track motion vectors in video, subtract background to detect moving objects.
- ๐ผ Professional Insight:
- Used in traffic monitoring (vehicle speed estimation), drone navigation (motion tracking), and sports analytics.
๐ MODULE 3: Advanced Topics in Computer Vision (Classes 13โ17)
Class 13: 3D Computer Vision & Depth Estimation
- ๐ Key Topics: Stereo vision & disparity mapping, Structure from Motion (SfM), 3D reconstruction techniques
- ๐ Assignment: Generate a point cloud from stereo images or an SfM pipeline.
- ๐ผ Professional Insight:
- 3D mapping essential in VR/AR, robotics (path planning), and architectural scanning.
Class 14: Neural Networks for Image Segmentation
- ๐ Key Topics: Semantic segmentation (UNet, DeepLab), instance segmentation (Mask R-CNN), medical imaging applications
- ๐ Assignment: Segment objects/cells using a deep network; evaluate IoU or dice score.
- ๐ผ Professional Insight:
- Medical domain relies heavily on accurate segmentation (tumor, organ boundaries).
- Instance segmentation used in robotic grasping (differentiating multiple objects).
Class 15: GANs & Image-to-Image Translation
- ๐ Key Topics: Generative Adversarial Networks (GANs), Pix2Pix, CycleGAN, neural style transfer, super-resolution
- ๐ Assignment: Train a CycleGAN to perform image translation (e.g., day โ night).
- ๐ผ Professional Insight:
- GANs power synthetic data generation, artistic style transfers, and upscaling for gaming/film industries.
Class 16: Vision Transformers (ViTs) & Self-Supervised Learning
- ๐ Key Topics: Attention mechanism, ViT vs. CNN, meta-learning, self-supervised pretraining
- ๐ Assignment: Fine-tune a small Vision Transformer model on a classification dataset; compare with CNN baseline.
- ๐ผ Professional Insight:
- ViTs represent cutting-edge research used by major AI labs.
- Self-supervised approaches reduce labeling costs in industrial or medical contexts.
Class 17: AI & Ethics in Computer Vision
- ๐ Key Topics: Bias & fairness in face recognition, explainability in deep learning models, privacy/surveillance concerns
- ๐ Assignment: Analyze potential bias in a face dataset; propose mitigation (data augmentation, balanced sampling).
- ๐ผ Professional Insight:
- Ethical considerations are paramount in facial recognition for law enforcement or HR.
- Regulatory frameworks (GDPR, HIPAA) demand explainability in medical or public surveillance use-cases.
๐ข MODULE 4: Research, Real-World Applications & Final Project (Classes 18โ20)
Class 18: Autonomous Vehicles & SLAM
- ๐ Key Topics: Lane detection, road scene understanding, visual odometry, LIDAR, SLAM
- ๐ Assignment: Explore a simple SLAM pipeline or lane detection using open-source datasets (KITTI, etc.).
- ๐ผ Professional Insight:
- SLAM is the backbone of robotics (warehouse bots) and self-driving (localization, obstacle avoidance).
- Lane/road detection vital for ADAS (driver assistance) in automotive industries.
Class 19: Final Project & Research Implementation
- ๐ Key Topics: Hands-on project (AI in healthcare, robotics, AR, object detection), OpenCV/TensorFlow/PyTorch coding, writing research reports
- ๐ Assignment: Build a pilot project end-to-end; optionally draft a short academic-style paper or extended abstract.
- ๐ผ Professional Insight:
- Mimics R&D cycles in industry or academiaโformulating problem, implementing solutions, documenting results.
- Strong reporting skills are crucial for stakeholder buy-in or research publications.
Class 20: Presentation & Future Research Directions
- ๐ Key Topics: Presenting research findings, future trends in AI & CV, preparing for publications/PhD
- ๐ Deliverable: Final project presentation, code/report submission, course reflection.
- ๐ผ Professional Insight:
- Skilled presentations help in pitching to investors, product demos, or technical conferences.
- Identifying next steps fosters lifelong learning and readiness for advanced roles in CV/AI.
7. In Depth Lesson Descriptions
Lesson 1: Digital Image Representation & Color Spaces
- ๐ Focus
- Image structure: pixels, resolution, bit depth
- Color models: RGB, HSV, YUV, YCbCr
- Compression formats: JPEG (lossy), PNG (lossless)
- ๐ป Coding Ideas
- Load & Compare: Load the same image in different color models (OpenCV stores as BGR by default), measure file sizes in JPEG vs. PNG.
- Histogram Visualization: Plot histograms (R, G, B channels) for a color image to observe distribution.
- ๐ Datasets
- Small set of color images (e.g., sample pictures from Kaggle or personal photo library).
- Consider using an image with distinct color regions to highlight color model differences.
- ๐ผ Professional Case Study
- Printing Industry: Ensuring accurate color reproduction in print media (magazines, posters).
- Web Streaming: Balancing file size (compression) and quality for e-commerce product images.
Lesson 2: Mathematical Foundations for Image Processing
- ๐ Focus
- Linear algebra basics (matrix ops, eigenvectors, PCA)
- Probability & statistics (Gaussian distribution for image noise)
- Convolution & filtering (kernel operations, correlation vs. convolution)
- ๐ป Coding Ideas
- Convolution Demo: Implement a custom convolution function (no built-in OpenCV filters).
- PCA on Images: Flatten images into vectors and apply PCA for dimensionality reductionโreconstruct images from principal components.
- ๐ Datasets
- A small grayscale image dataset (e.g., MNIST or fashion MNIST) for PCA demonstration.
- Noisy images (Gaussian noise added) to illustrate distribution assumptions.
- ๐ผ Professional Case Study
- Quality Control: Using convolution-based filters to reduce noise in manufacturing line scans.
- Face Recognition: Early systems using PCA (Eigenfaces) to reduce dimensionality before classification.
Lesson 3: Geometric Transformations & Image Warping
- ๐ Focus
- Translation, rotation, scaling
- Affine & perspective transforms
- Homography & stitching (panoramas)
- ๐ป Coding Ideas
- Manual Warping: Apply an affine transformation matrix to rotate an image by a given angle.
- Panorama Stitching: Use OpenCVโs
findHomography
andwarpPerspective
to stitch overlapping images.
- ๐ Datasets
- Overlapping scenic images (e.g., city skyline) or campus photos for stitching.
- Synthetic shapes (rectangles) to demonstrate transformations clearly.
- ๐ผ Professional Case Study
- Augmented Reality: Overlaying virtual objects on planar surfaces, requiring accurate homography.
- Drone Mapping: Aligning aerial images for large area coverage or mosaic creation.
Lesson 4: Spatial & Frequency Domain Processing
- ๐ Focus
- Filters in spatial domain (Gaussian/median/bilateral)
- Frequency domain transforms (DFT/FFT), high-pass & low-pass filters
- Discrete Cosine Transform (DCT) for compression
- ๐ป Coding Ideas
- Compare Filters: Implement and compare noise reduction with median vs. Gaussian vs. bilateral on the same noisy image.
- FFT-based Filters: Visualize the frequency spectrum of an image, apply a circular low-pass filter, inverse transform.
- ๐ Datasets
- Images with different types of noise (salt & pepper, Gaussian).
- Possibly a standard test image (e.g.,
lenna.png
,cameraman.tif
) for frequency analysis.
- ๐ผ Professional Case Study
- Surveillance: Frequency filters used to remove periodic camera noise or flicker from fluorescent lighting.
- Mobile Apps: DCT integral to JPEG compression, optimizing image storage/transmission in social media.
Lesson 5: Edge Detection & Image Segmentation
- ๐ Focus
- Edge detection: Sobel, Prewitt, Canny
- Thresholding: Otsu, adaptive methods
- Region-based segmentation: Watershed, graph cuts
- ๐ป Coding Ideas
- Canny Edge Tuner: Interactively adjust thresholds for Canny in a Jupyter widget to see real-time changes.
- Watershed Segmentation: Segment a grayscale image (e.g., coins on a uniform background).
- ๐ Datasets
- Simple scenes with clear edges (coins, shapes).
- More complex images (e.g., cell clusters for watershed).
- ๐ผ Professional Case Study
- Barcode/QR Scanning: Reliable edge detection critical in reading codes.
- Medical: Identifying organ boundaries (segmentation) in CT/MRI scans.
Lesson 6: Morphological Image Processing
- ๐ Focus
- Erosion, dilation, opening, closing
- Convex hull, skeletonization
- Connected component labeling (counting objects)
- ๐ป Coding Ideas
- Shape Extraction: Remove noise or small artifacts with opening, fill holes with closing.
- Connected Components: Label distinct objects in a binary image, compute area/perimeter.
- ๐ Datasets
- Binary images with noise (e.g., scanned text, thresholded shapes).
- Industrial scenarios (conveyor belt images with multiple items).
- ๐ผ Professional Case Study
- Manufacturing: Distinguishing defective parts from background by morphological ops.
- Handwriting Recognition: Skeletonization to trace letters, reduce them to minimal strokes.
Lesson 7: Feature Detection & Extraction
- ๐ Focus
- Corner detection (Harris, FAST), keypoint descriptors (SIFT, SURF, ORB, BRIEF, FREAK)
- HOG (Histogram of Oriented Gradients)
- ๐ป Coding Ideas
- Compare Keypoints: Evaluate SIFT vs. ORB on a small image set for speed vs. robustness.
- HOG Visualization: Show gradient magnitude/orientation in blocks for a simple image.
- ๐ Datasets
- Scenes with distinct corners/features (buildings, patterns).
- Cars or pedestrians for HOG-based detection.
- ๐ผ Professional Case Study
- SLAM: Relying on robust keypoints for mapping unknown environments in robotics.
- Security: HOG descriptors in classical person detection solutions (before deep learning).
Lesson 8: Feature Matching & Object Recognition
- ๐ Focus
- Brute-force vs. FLANN matching
- Bag of Visual Words (BoVW) approach
- Template matching basics
- ๐ป Coding Ideas
- Local Feature Matching: Detect features in two images of the same scene from different angles, match, and compute homography.
- BoVW Mini-Project: Classify a small dataset (e.g., logos) using BoVW representation.
- ๐ Datasets
- Logo images, toy objects from multiple viewpoints.
- Print media scans (magazine ads) for template matching.
- ๐ผ Professional Case Study
- E-commerce: Logo detection to track brand presence.
- Robotics: Identifying tools or known objects by template or feature-based methods.
Lesson 9: Machine Learning for Image Classification
- ๐ Focus
- Traditional ML: SVM, k-NN, Decision Trees
- PCA & LDA for feature reduction
- Feature engineering for images
- ๐ป Coding Ideas
- SVM Classifier: Use HOG or raw pixels, train/test on a small dataset (e.g., cats vs. dogs).
- Dimensionality Reduction: Apply PCA, see how classification accuracy changes.
- ๐ Datasets
- Simple binary classification sets (cats/dogs, MNIST subsets).
- Possibly extend to multi-class if time/resources permit.
- ๐ผ Professional Case Study
- Embedded or low-power devices: Traditional ML models can be smaller/faster than deep networks.
- Medical or specialized fields with limited data: SVM + PCA might suffice.
Lesson 10: Deep Learning for Image Processing
- ๐ Focus
- Convolutional Neural Networks (CNNs)
- Transfer learning (ResNet, MobileNet)
- Implementation with TensorFlow or PyTorch
- ๐ป Coding Ideas
- Transfer Learning: Fine-tune MobileNet on a custom dataset (small specialized domain).
- CNN from Scratch: Build a small CNN for digit classification (MNIST).
- ๐ Datasets
- CIFAR-10 or a custom curated dataset relevant to the class.
- Kaggle sets (e.g., โDog vs. Catโ if licenses permit).
- ๐ผ Professional Case Study
- Industry Standard: CNNs for image classification, object detection on large-scale data.
- Healthcare: Transfer learning often used when labeled data is scarce.
Lesson 11: Object Detection & Tracking
- ๐ Focus
- Classical detection (Haar Cascades, HOG+SVM) vs. modern (YOLO, SSD, Faster R-CNN)
- Tracking algorithms (Kalman Filter, Mean-Shift, DeepSORT)
- ๐ป Coding Ideas
- Realtime Detection: Implement YOLOv5 (or a classic HOG+SVM) in a live webcam feed, measure FPS.
- Tracking: Track an object across frames with Mean-Shift or Kalman Filter.
- ๐ Datasets
- Street scenes for detecting cars/pedestrians.
- Surveillance footage or traffic camera clips.
- ๐ผ Professional Case Study
- Smart City: Pedestrian detection for safety, vehicle tracking for traffic analysis.
- Retail: People counting, shelf analytics in real-time.
Lesson 12: Optical Flow & Motion Analysis
- ๐ Focus
- Lucas-Kanade, Farneback optical flow
- Background subtraction (MOG2, KNN)
- Applications in surveillance/autonomous vehicles
- ๐ป Coding Ideas
- Optical Flow Demo: Compute flow vectors on a short video, visualize them as arrows.
- Moving Object Detection: Combine background subtraction with flow to track a single object.
- ๐ Datasets
- Short dynamic video: walking people, moving vehicles.
- Could use popular optical flow benchmarks like Kitti or Middlebury.
- ๐ผ Professional Case Study
- Traffic: Speed measurement, counting vehicles in highways or toll booths.
- Robotics: Estimating self-motion (egomotion) in drones.
Lesson 13: 3D Computer Vision & Depth Estimation
- ๐ Focus
- Stereo vision & disparity mapping
- Structure from Motion (SfM) for 3D reconstruction
- Depth sensors (RGB-D) basics
- ๐ป Coding Ideas
- Stereo Matching: Compute disparity map using two camera images (rectified pair).
- SfM: Use an open-source library (OpenCV or COLMAP) to reconstruct sparse 3D from multiple views.
- ๐ Datasets
- Stereo image pairs (e.g., KITTI dataset or self-captured stereo rig).
- Multi-view pictures of a small object to generate a 3D model.
- ๐ผ Professional Case Study
- Autonomous Driving: Depth estimation for obstacle avoidance.
- AR/VR: 3D environment reconstruction for immersive experiences.
Lesson 14: Neural Networks for Image Segmentation
- ๐ Focus
- Semantic segmentation (UNet, DeepLab)
- Instance segmentation (Mask R-CNN)
- Medical imaging (tumor detection, organ segmentation)
- ๐ป Coding Ideas
- UNet: Implement or fine-tune a UNet on a small dataset (cells, roads).
- Mask R-CNN: Demo for instance segmentation of multiple objects in a scene.
- ๐ Datasets
- Medical: e.g., open-source CT or MRI scans (liver, lung).
- Street scenes: detect cars, pedestrians, roads.
- ๐ผ Professional Case Study
- Healthcare: Automatic tumor detection or organ segmentation to reduce manual radiology work.
- Self-driving: Scene parsing (roads, sidewalks, vehicles) for advanced autonomy.
Lesson 15: GANs & Image-to-Image Translation
- ๐ Focus
- Generative Adversarial Networks (GANs), Pix2Pix, CycleGAN
- Neural style transfer, super-resolution
- ๐ป Coding Ideas
- CycleGAN: Translate images from day to night or horse to zebra.
- Super-Resolution: Implement a basic SRGAN or ESRGAN module for upscaling low-res images.
- ๐ Datasets
- Domain-specific pairs (day โ night, summer โ winter).
- Low-res vs. high-res image pairs for super-resolution.
- ๐ผ Professional Case Study
- Entertainment: Photo style transfer, game asset generation.
- E-commerce: Super-resolution of product images to enhance user experience.
Lesson 16: Vision Transformers (ViTs) & Self-Supervised Learning
- ๐ Focus
- Attention mechanism, ViT vs. CNN
- Self-supervised pretraining, meta-learning
- ๐ป Coding Ideas
- ViT Fine-tuning: Use a pretrained ViT model on a custom classification dataset, compare results to a CNN baseline.
- Self-Supervised: Experiment with a small self-supervised approach (e.g., rotating images as pseudo-labels).
- ๐ Datasets
- Standard classification sets (CIFAR-10, ImageNet mini-subset).
- Self-collected images for smaller domain tasks.
- ๐ผ Professional Case Study
- Cutting-Edge Research: ViTs are used by major AI labs for high accuracy in image tasks.
- Data-Limited Scenarios: Self-supervised learning mitigates label scarcity in specialized industries (e.g., manufacturing anomalies).
Lesson 17: AI & Ethics in Computer Vision
- ๐ Focus
- Bias & fairness in face recognition
- Explainability in deep learning (Grad-CAM, saliency)
- Privacy & surveillance issues
- ๐ป Coding Ideas
- Bias Analysis: Take a small face dataset, test a face recognition model for demographic bias.
- Explainability: Use Grad-CAM on a CNN-based classifier to see which regions influence decisions.
- ๐ Datasets
- Public face dataset with diversity (UTKFace, FairFace).
- Could use simpler classification sets for interpretability demos.
- ๐ผ Professional Case Study
- Legal: Some regions ban or heavily regulate face recognition (GDPR, local laws).
- Corporate: Ensuring fairness to prevent brand damage or lawsuits if biases are found.
Lesson 18: Autonomous Vehicles & SLAM
- ๐ Focus
- Lane detection, road scene understanding
- Visual odometry, LIDAR integration
- Simultaneous Localization & Mapping (SLAM)
- ๐ป Coding Ideas
- Lane Detection: Use edge detection + Hough transforms or a CNN-based approach on a dashcam dataset.
- SLAM Demo: Briefly explore a library like ORB-SLAM or RTAB-Map in a simulation environment.
- ๐ Datasets
- Public driving datasets (KITTI, Udacity Self-driving Car).
- Or synthetic environment from a simulator (CARLA).
- ๐ผ Professional Case Study
- Self-Driving: Core modules for lane following, obstacle detection, path planning.
- Robotics: Warehouse robots employing SLAM for dynamic mapping and navigation.
Lesson 19: Final Project & Research Implementation
- ๐ Focus
- Hands-on project in a chosen domain (healthcare, robotics, AR, object detection)
- Implementation details (OpenCV, TensorFlow, PyTorch)
- Writing research reports & academic papers (optional)
- ๐ป Coding Ideas
- Capstone: Students/teams select a dataset, implement a pipeline (preprocessing โ model โ evaluation).
- Report: Draft a short paper-style or blog post describing methodology, results, lessons learned.
- ๐ Datasets
- Domain-specific: medical images, automotive scenes, AR markers, etc.
- Kaggle or open repositories if relevant.
- ๐ผ Professional Case Study
- Industry: Project-based deliverables mirror real R&D cyclesโdefining scope, iterating on prototypes, final presentation.
- Academia: Encourages systematic documentation, critical for future publications.
Lesson 20: Presentation & Future Research Directions
- ๐ Focus
- Presenting final projects, Q&A
- Emerging trends (3D CV, neural radiance fields, large-scale self-supervised vision)
- Preparing for publications, PhD programs, advanced career paths
- ๐ Deliverable
- Final project presentation/demo
- Course feedback or post-course survey
- ๐ผ Professional Insight
- Pitching data science or CV solutions to stakeholders/investors is a vital skill.
- Understanding future directions ensures students remain adaptable in fast-evolving CV research areas.
8. Assessment & Grading
-
๐ Weekly/Regular Assignments (40%)
- ๐ Coding tasks, problem sets, short reflections.
- Reinforces theoretical and practical components.
-
๐ Quizzes (10%)
- ๐ Occasional quizzes (announced or pop).
- Covers core image processing & vision concepts.
-
๐ผ Capstone Project (40%)
- ๐ Real-world pipeline: from data gathering/pre-processing โ advanced modeling โ final report/demonstration.
- Demonstrates integrated skills from the entire course.
-
๐ค Participation (10%)
- ๐ Active involvement in Zoom sessions, breakout discussions, Q&A.
- Peer reviews and collaboration simulate professional team settings.
Grade Scale
- A = 90โ100%
- B = 80โ89%
- C = 70โ79%
- D = 60โ69%
- F = < 60%
9. Course Policies
-
๐ท๏ธ Attendance & Engagement
- Attend and contribute in Zoom sessions.
- Notify absences in advance when possible.
-
๐ข Communication
- Official notices & announcements via email.
- For help, contact hello@softwareintelligence.ai.
-
โฑ๏ธ Late Submissions
- Late work may incur penalties unless previously arranged.
- Extension requests should be made ahead of deadlines.
-
โ ๏ธ Academic Integrity
- Plagiarism & unauthorized collaboration are prohibited.
- Violations follow institutional regulations.
-
โ๏ธ Technical Preparedness
- Ensure Python/OpenCV environment is installed and tested.
- Familiarity with Zoom features (screen share, chat) recommended.
10. Final Note
Welcome to Mastering in Image Processing and Computer Vision! Over 20 lessons (total 40 hours 20 minutes), youโll learn the full pipelineโfrom classic filtering and segmentation to advanced AI methods like GANs and vision transformers. Remember:
- Practice continuously with real or synthetic datasets.
- Collaborate with peersโfeedback accelerates learning.
- Experiment with new libraries (OpenCV, PyTorch) to gain industry-ready skills.
We look forward to an engaging and hands-on semester exploring the power of computer vision!
๐จโ๐ซ Instructor: Mejbah Ahammad
โ๏ธ Contact: +8801874603631
๐ Website: http://softwareintelligence.ai/
๐ง Email: hello@softwareintelligence.ai
(C) 2025 Software Intelligence & Intelligence Academy โ All Rights Reserved.